What Algorithms Say About You
Artificial intelligence is letting us make predictive algorithms that translate languages and spot diseases as well or better than humans. But these systems are also being used to make decisions about hiring and criminal sentencing. Do computers trained on vast datasets of human experience learn human biases, like sexism and racism? Is it possible to create an algorithm that is fair for everyone? And should you have the right to know when these algorithms are being used and how they work?
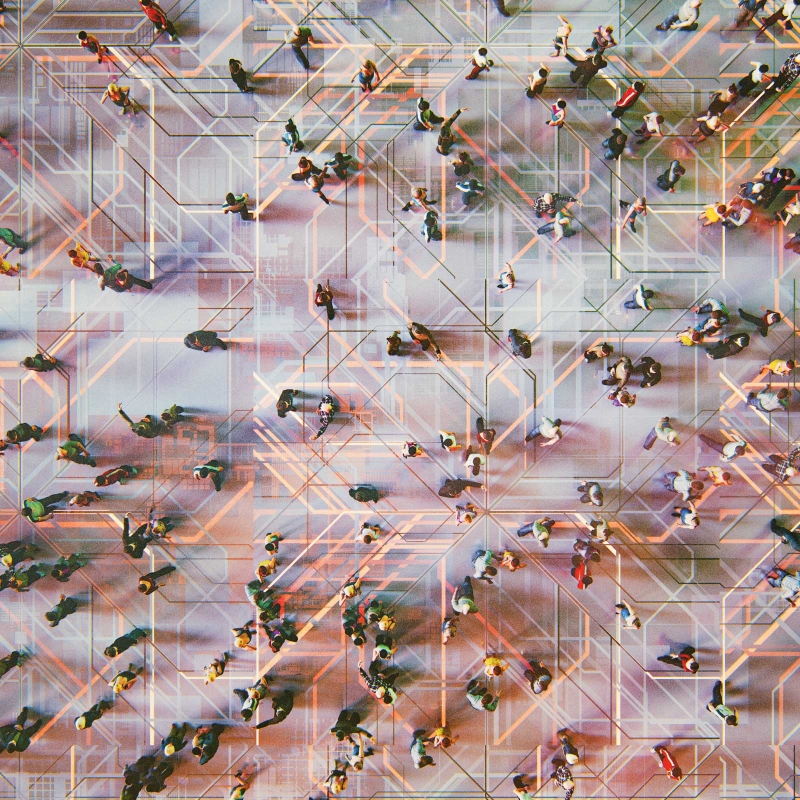
Guests
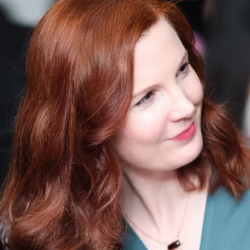
Kate Crawford
Co-Founder,
AI Now Institute
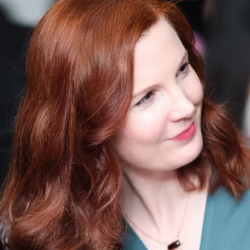
Kate Crawford has spent over a decade researching the social, political, and environmental implications of artificial intelligence. She is a Distinguished Research Professor at NYU, co-founder of the AI Now Institute, a Senior Principal Researcher at Microsoft Research, and the inaugural visiting Chair in AI and Justice at the École Normale Supérieure in Paris. Kate is also an electronic musician who is known for her work in the bands B(if)tek and Metric Systems. Her forthcoming book is called Atlas of AI (Yale, 2021).
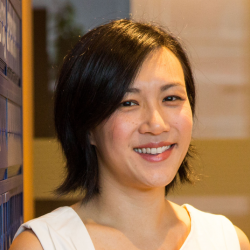
Lily Peng
Product Manager,
Google Research
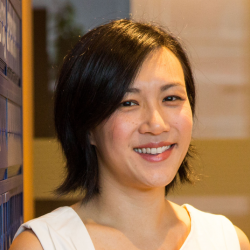
Lily Peng is a physician-scientist, with an M.D. and Ph.D. from University of California, San Francisco, who works to bring breakthrough science from bench to bedside. She is the lead for the Medical Imaging team for Google AI Healthcare, which focuses on applying deep learning to medical data. Before Google, she was a product manager at an online networking service for physicians, and a co-founder of a medical device start-up developing a small implantable drug delivery device.
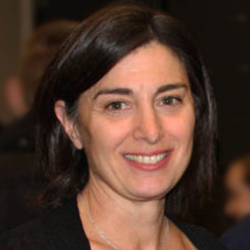
Christine Vogeli
Director of Evaluation and Research,
Partners Center for Population Health
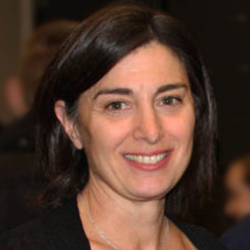
Christine Vogeli is a scientist and health services researcher whose interests include health disparities, healthcare quality, and primary care. On the faculty of the Harvard Medical School, she serves as director of evaluation and research at the Center of Population Health at Mass General Brigham in Boston. She has played a leading role in the design, implementation and evaluation of care delivery innovations across the Mass General Brigham integrated health care system.
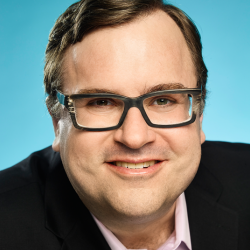
Reid Hoffman
Co-Founder, LinkedIn & Partner,
Greylock
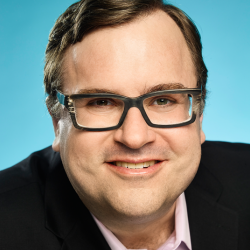
An accomplished entrepreneur, executive, and investor, Reid Hoffman has played an integral role in building many of today’s leading consumer technology businesses. He is the co-author of three best-selling books: The Start-Up of You, The Alliance, and Blitzscaling. He is the host of Masters of Scale, an original podcast series and the first American media program to commit to a 50-50 gender balance for featured guests.
Photo credit: David Yellen
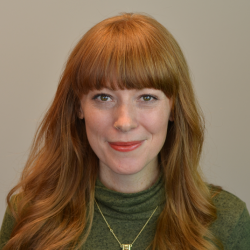
Lindsey Zuloaga
Director of Data Science,
HireVue
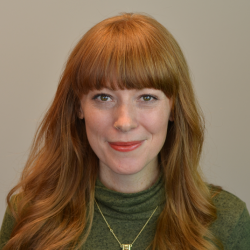
Dr. Lindsey Zuloaga is the Director of Data Science at HireVue, managing a team that builds and validates machine learning algorithms to predict job-related outcomes. Lindsey has a Ph.D. in Applied Physics and started her career as a Data Scientist in the healthcare space. At HireVue, she is working to completely transform traditional interviewing with a platform that focuses on understanding more of the candidate as a whole person, including interview responses, coding abilities, and cognitive skills as opposed to just the facts shown on a resume.
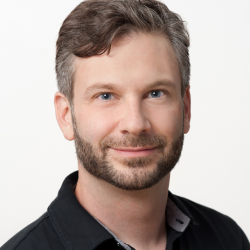
Greg Corrado
Principal Scientist, Google Research & Co-Founder,
Google Brain Team
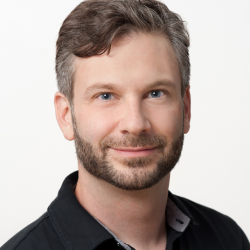
Greg Corrado is currently a principal scientist and research director at Google AI, where he is co-founder of the Google Brain team. He works at the intersection of biological neuroscience, artificial intelligence, and scalable machine learning and has published in fields ranging across behavioral economics, neuromorphic device physics, systems neuroscience, and deep learning. Before coming to Google, he worked at IBM Research on neuromorphic silicon devices and large-scale neural simulations.
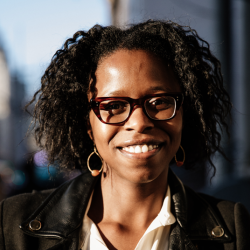
Rashida Richardson
Visiting Scholar, Rutgers Law School & Senior Fellow,
Digital Innovation and Democracy Initiative at the German Marshall Fund
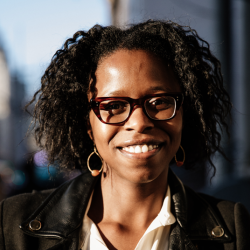
Rashida Richardson is a civil rights lawyer specializing in race, emerging technologies and the law. She is a Visiting Scholar at Rutgers Law School and a Senior Fellow in the Digital Innovation and Democracy Initiative at the German Marshall Fund. Richardson studies the social and civil rights implications of data-driven technologies, including artificial intelligence. Previously, Richardson served as the director of policy research at New York University’s AI Now Institute and legislative counsel at the American Civil Liberties Union of New York.
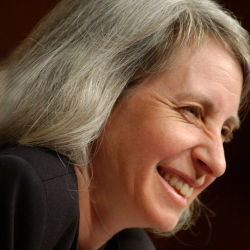
Martha Minow
300th Anniversary University Professor,
Harvard Law School
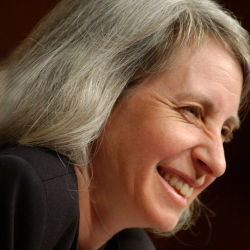
Martha Minow served as Dean of the Harvard Law School from 2009 to 2017. An expert in human rights and advocacy for members of racial and religious minorities and for women, children, and persons with disabilities, Minow writes and teaches about privatization, military justice, and ethnic and religious conflict. For the past several years, she has taught law and computer science students about emerging issues of fairness, privacy, and accountability in the age of algorithms. She has been on the faculty of Harvard University since 1981, where she is currently 300th Anniversary University Professor.
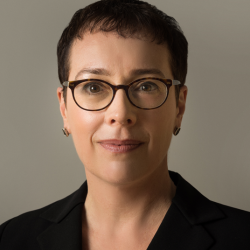
Julia Angwin
Editor-in-Chief,
The Markup
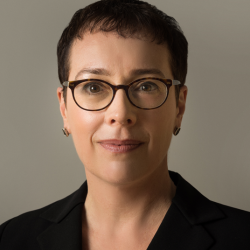
Julia Angwin is an award-winning investigative journalist who uses data-centered journalism to explore technology and the people affected by it. She is editor-in-chief and founder of The Markup and has led investigative teams at ProPublica and The Wall Street Journal. She is the author of Dragnet Nation: A Quest for Privacy, Security and Freedom in a World of Relentless Surveillance, (Times Books, 2014) and Stealing MySpace: The Battle to Control the Most Popular Website in America (Random House, March 2009).
Photo credit: Rinze Van Brug
Featured in the Boston Globe
Predictions—whether algorithmic or human—may not be fair
An OpEd by Sharad Goel, Julian Nyarko, and Roseanna Sommers.
Who am I to decide when algorithms should make important decisions?
An OpEd by Meredith Whittaker, a cofounder of AI Now Institute.
Referenced in the Episode
A 2016 story from The New York Times about how Google used artificial intelligence to transform Google Translate.
Building High-level Features Using Large Scale Unsupervised Learning
Google’s famous 2012 paper about it’s newly developed cat-identifying neural network.
Google’s website dedicated to their deep learning diabetic retinopathy project.
Amazon Scraps Secret AI Recruiting Tool That Showed Bias Against Women
A 2018 story from Reuters about how Amazon’s hiring tool propagated systemic bias against women.
Dissecting Racial Bias in an Algorithm Used to Manage the Health of Populations.
A 2019 study published in Science dissecting racial bias in a commercial algorithm used to predict which patients would benefit most from a high-risk care management program. Guest Dr. Christine Vogeli is a co-author.
Machine Bias: There’s software used across the country to predict future criminals. And it’s biased against blacks
ProPublica’s investigation into how algorithmic bias shaped COMPAS sentencing software, written by guest Julia Angwin and colleagues.
Technical Flaws of Pretrial Risk Assessments Raise Grave Concerns
An open statement of concern from 27 prominent researchers, including guest Martha Minow, regarding the use of pretrial risk assessment as a means of lowering pretrial jail populations.
Lunchtime Leniency: Judges' Rulings Are Harsher When They Are Hungrier
A Scientific American article that reports on a 2011 study from researchers at Ben Gurion University in Israel and Columbia University that found judges granted far more requests at the beginning of the day’s session and almost none at the end (the approvals jumped back up right after a snack break, though).
2016 Wisconsin Supreme Court decision written by Justice Ann Walsh Bradley.
Reducing Bias and Widening the Candidate Pool: Why We Built HireVue Assessments.
HireVue CTO Loren Laren’s series breaking down the company’s claim that machine learning can eliminate unconscious human bias.
Gender Shades: Intersectional Accuracy Disparities in Commercial Gender Classification
A 2018 study from MIT researcher Joy Buolamwini and Timnit Gebru (now a research scientist at Google), which found programs from major technology companies demonstrate both skin-type and gender biases.
EPIC Files Complaint with FTC about Employment Screening Firm HireVue
The Electronic Privacy Information Center’s 2019 complaint against HireVue.
New York City Automated Decision Systems Task Force Report
This report, published in November 2019, is the product of New York City’s task force to provide recommendations regarding government use of automated decision systems. New York City was the first US jurisdiction to enact a law creating a task force of this kind.
Confronting Black Boxes: A Shadow Report on The New York City Automated Decision Task Force
Guest Rashida Richardson authored this shadow report written in anticipation of the New York City Automated Decision Task Force Report.
Idaho Bill No. 118
In 2019, Idaho passed a first-in-the-nation law requiring that pre-trial risk algorithms be free of bias and transparent.
Algorithmic Accountability Act
A bill introduced in the US Congress which would require that private companies ensure certain types of algorithms are audited for bias.
Further Learning
How to Develop Machine Learning Models for Healthcare
A 2019 piece from Nature on the implementation of machine learning models in healthcare.
Kate Crawford’s keynote speech for the Neural Information Processing Systems 2017 Conference.
AI Now Reports
AI Now’s 2019 annual reports on the social implications of artificial intelligence, including recommendations to address the ways that AI widens inequity and to help promote algorithmic accountability.
When the Robot Doesn’t See Dark Skin
A New York Times op-ed on algorithmic bias from MIT’s Joy Buolamwini.
Can you make AI fairer than a judge? Play our courtroom algorithm game
An interactive article from the MIT Technology Review where readers can play a game to improve the COMPAS algorithm.
21 fairness definitions and their politics
Princeton Professor Arvind Narayanan’s talk at FAT* 2018.
Fairness and Machine Learning: Limitations and Opportunities
An online textbook from Solon Barocas, Moritz Hard, and Avind Narayanan.
A 2016 Harvard Law Review piece summarizing the Loomis case argues that Wisconsin Supreme Court ruling is insufficient.
Why 'Ditch the algorithm' is the future of political protest
Due to the COVID-19 pandemic, A-levels (UK high school exams) were canceled and replaced by teacher assessments and an algorithm. This 2020 opinion piece, written in response to the uproar that ensued, considers the issues with using predictive algorithms and ethical decision making.